Generative Ai and Privacy
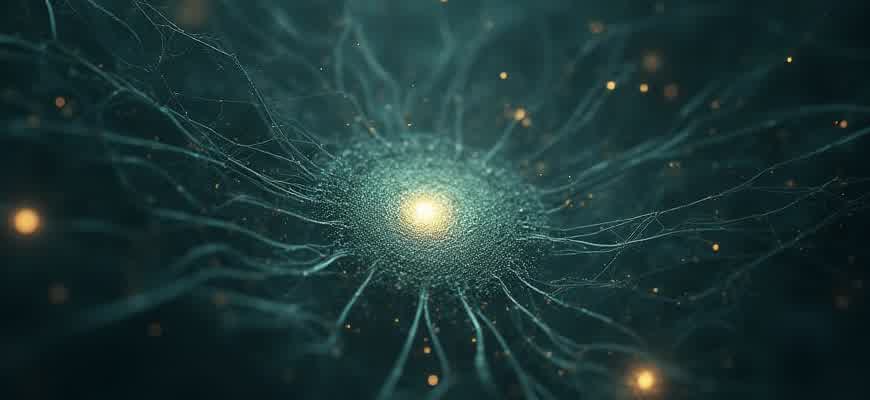
Generative AI systems, capable of creating content such as text, images, and even audio, have raised significant concerns regarding user privacy and data protection. These models often require vast amounts of personal data to train, which can lead to unintended exposure of sensitive information. As these technologies evolve, understanding the risks associated with data usage is critical for both developers and end users.
Key Issues in Data Privacy with Generative AI:
- Data Breach Risks: AI models can unintentionally memorize and regurgitate private data encountered during training, putting personal information at risk.
- Lack of Transparency: Many AI models are considered “black boxes,” meaning the data they use and how it’s processed may not be easily understood or audited.
- Consent and Control: Users may unknowingly provide consent for their data to be used in AI development without fully understanding the scope or purpose.
Important Considerations:
"As AI models continue to grow more complex, the line between legitimate use of data and potential privacy violations becomes increasingly difficult to draw."
The responsibility of developers to implement safeguards against privacy violations is paramount. Without appropriate measures, the risk of AI systems unintentionally exploiting or mishandling private data remains a serious concern.
Generative AI and Privacy: Addressing Key Challenges and Solutions
As generative AI technologies advance, they introduce new risks to personal privacy that need to be carefully addressed. With the ability to create content that closely mimics real data, these systems raise concerns about unauthorized use of sensitive information. The key challenge lies in balancing the innovation of AI-generated outputs with safeguarding individual privacy rights, particularly when AI systems are trained on large datasets containing personal information.
To effectively manage privacy risks, it is crucial to implement strategies that limit the exposure of personal data during the training and deployment of AI models. By integrating privacy-focused techniques such as differential privacy and federated learning, we can reduce the likelihood of privacy breaches while still benefiting from the capabilities of generative AI.
Key Challenges in Privacy with Generative AI
- Data Leakage: AI systems may inadvertently memorize and reproduce personal data from training sets, leading to the risk of data leakage in generated content.
- Bias and Discrimination: If AI models are trained on biased datasets, they may produce outputs that reflect and perpetuate harmful stereotypes or discriminatory practices.
- Lack of Transparency: The black-box nature of many generative models makes it difficult to track how personal data is used and ensure compliance with privacy regulations.
Solutions to Protect Privacy
- Differential Privacy: This technique adds controlled noise to the training data, making it impossible to trace any individual’s data in the output, thereby preventing leakage.
- Federated Learning: In this approach, AI models are trained across decentralized devices, keeping sensitive data on local machines and only sharing model updates, minimizing the risk of data exposure.
- Data Anonymization: Ensuring that personally identifiable information (PII) is removed from datasets before they are used for training can significantly reduce privacy concerns.
Privacy Protection Measures in Practice
Privacy Measure | Description | Impact on Privacy |
---|---|---|
Differential Privacy | Adding noise to data during training to prevent identification of individual data points. | High protection, maintains statistical accuracy without compromising privacy. |
Federated Learning | Decentralized training where data remains on the device and only aggregated model updates are shared. | Minimizes data exposure and keeps sensitive information local. |
Data Anonymization | Removing or masking personal identifiers from datasets before training. | Reduces privacy risks by ensuring data cannot be traced back to individuals. |
"The key to ensuring privacy in generative AI lies in not only the technology itself but also in the ethical frameworks we establish around its use."
How Generative AI Influences Data Privacy in Practical Use Cases
Generative AI technologies have revolutionized the way data is processed, but they also raise significant concerns regarding privacy. These systems can generate highly realistic content such as images, text, and even synthetic data based on training sets, which often include sensitive personal information. This raises a host of questions about how such AI models handle, store, and potentially expose private data in real-world applications.
The impact of generative models on data privacy is particularly relevant in industries such as healthcare, finance, and entertainment, where sensitive personal data is frequently utilized. As these AI systems are trained on massive datasets, there is a potential for unintentional exposure of private information, posing serious privacy risks if not properly managed.
Key Privacy Concerns
- Data Leakage – Generative AI can inadvertently produce content that includes private or confidential data, such as names, medical details, or financial information.
- Re-identification Risks – Even anonymized data used for training can be re-identified when processed by advanced generative models, compromising user privacy.
- Data Ownership – In many cases, the individuals whose data is used to train generative AI models may not be aware of or consent to how their information is being utilized.
Strategies to Mitigate Privacy Issues
- Data Anonymization – Techniques such as differential privacy can help ensure that sensitive data cannot be traced back to individual users.
- Secure Data Handling – Ensuring that datasets are encrypted and securely managed can reduce the likelihood of unauthorized access or leakage.
- Transparency and Consent – Clear policies on how personal data is used, coupled with obtaining informed consent, can foster trust and reduce privacy violations.
“Generative AI has the potential to reshape industries, but it also introduces substantial privacy concerns that must be addressed to maintain trust and compliance with data protection regulations.”
Real-World Examples
Industry | Privacy Challenge | Mitigation Strategies |
---|---|---|
Healthcare | Generation of synthetic patient data can inadvertently reveal private health details. | Implementing differential privacy techniques in training models. |
Finance | AI-generated financial reports may contain sensitive customer information. | Strict encryption and anonymization of client data used in training. |
Entertainment | AI tools may generate content using personal data such as images and voices. | Ensuring opt-in consent and limiting data access for training purposes. |
Managing User Consent and Data Usage in AI Models
In the age of generative AI, managing user consent and the appropriate usage of personal data is crucial for maintaining trust and ensuring compliance with privacy laws. AI systems require vast amounts of data to function effectively, but users must be informed and in control of how their data is collected, processed, and utilized. Failure to manage this process properly can lead to violations of privacy, legal consequences, and significant damage to an organization's reputation.
Consent management must go beyond a simple opt-in mechanism. It involves clear communication of what data will be used, for what purpose, and how long it will be retained. Implementing transparency and offering users granular control over their data can help organizations build trust with their audience and stay aligned with data protection regulations.
Key Considerations in Managing Consent
- Transparency: Users must understand exactly how their data will be used by AI models.
- Granular Control: Offering options for users to manage their consent on a per-use case basis.
- Data Retention Policies: Defining clear retention periods and ensuring data is deleted when no longer needed.
- Revocation of Consent: Providing users the option to withdraw their consent at any time.
Steps for Proper Consent Collection
- Clear and Accessible Information: Provide concise, understandable explanations of how data will be used.
- Opt-in Mechanisms: Ensure users actively give consent rather than assuming agreement.
- Audit Trails: Track when and how consent is given or revoked for transparency.
- Periodic Re-consent: Regularly ask users to reaffirm their consent, especially when data usage practices change.
Data Usage and AI Model Training
AI models often require training on diverse datasets, which can include sensitive information. Ensuring that user data is anonymized and aggregated when used for training purposes is essential to protect individual privacy. Organizations must also monitor and enforce policies regarding third-party access to data, ensuring that only authorized entities have access to sensitive user information.
Important: Compliance with data protection regulations such as GDPR or CCPA is mandatory for any organization using AI models to process personal data.
Action | Benefit |
---|---|
Transparent Data Policies | Builds user trust and ensures regulatory compliance. |
Granular User Control | Enhances user experience and privacy protection. |
Regular Consent Updates | Ensures ongoing compliance and up-to-date user preferences. |
Ensuring Compliance with GDPR and Other Privacy Regulations
As generative AI models become increasingly integral to various industries, ensuring compliance with privacy regulations like GDPR (General Data Protection Regulation) and other regional laws is crucial. These regulations set stringent guidelines for how personal data is handled, processed, and stored. Organizations must navigate these requirements carefully to avoid penalties and maintain user trust.
Privacy concerns in AI applications stem from their ability to process vast amounts of sensitive information. Therefore, organizations deploying these technologies must implement robust measures to guarantee that personal data is protected at all stages of data processing, from collection to storage and use in AI model training.
Key Strategies for Compliance
- Data Minimization: Collect only the data necessary for the specific purpose, avoiding excessive or irrelevant information.
- Transparency: Inform users clearly about data usage, processing purposes, and AI system interactions, ensuring informed consent.
- Data Anonymization: Anonymize personal data where possible to prevent identification, reducing privacy risks.
- Data Access Controls: Implement strict access controls and audit trails to monitor who accesses sensitive data and why.
- Data Subject Rights: Enable users to exercise their rights to access, correct, or delete their personal data at any time.
"Organizations must build privacy and security into AI systems from the design phase, not just as an afterthought."
Compliance Measures for AI Data Usage
- Conduct Regular Privacy Impact Assessments (PIAs): Ensure that potential risks to personal data are identified and mitigated at every stage of AI model development.
- Ensure Data Residency Compliance: Be aware of data storage locations and ensure that data is processed in accordance with regional regulations, especially in jurisdictions with stricter laws.
- Update AI Model Training Practices: Train AI models using datasets that comply with privacy laws, ensuring that any personal data used is processed lawfully.
- Ensure User Consent: Obtain explicit consent from users for data usage, especially in AI systems that rely on sensitive data categories like health or biometric data.
GDPR Compliance Checklist for AI Systems
Action | Status |
---|---|
Data Minimization | ✔️ |
Informed Consent from Users | ✔️ |
Data Anonymization | ❌ |
Transparency in Data Processing | ✔️ |
Data Access Controls | ✔️ |
Securing Sensitive Information in AI-Generated Content
As AI models become more integrated into content creation, securing sensitive information has emerged as a critical concern. AI-generated content, whether text, images, or videos, may inadvertently include private or classified data that could be exploited. The challenge is not only ensuring that these models do not leak such information but also guaranteeing that any generated output does not expose it to unintended audiences. Effective mechanisms for protecting sensitive information are vital to maintaining trust in AI-driven applications.
To prevent privacy breaches, several strategies are being developed to limit AI’s access to confidential data. These methods range from technical safeguards in model training to more robust post-processing measures for filtering and monitoring output. Below, we explore a few key strategies employed to secure private information in AI-generated content.
Key Strategies for Securing Sensitive Data in AI Outputs
- Data Anonymization: AI models can be trained to strip sensitive identifiers such as names, addresses, or phone numbers from the data before it is processed. This ensures that even if the model generates content based on personal data, no identifiable information is exposed.
- Controlled Output Generation: Through fine-tuning models and restricting certain prompts or input parameters, it is possible to prevent the generation of sensitive content by setting clear boundaries on what can and cannot be produced.
- Post-Generation Monitoring: After content is generated, automated systems can analyze it for the presence of private or protected information. If such information is found, it can either be redacted or flagged for review.
Challenges and Future Considerations
While these measures help reduce the risk of privacy violations, they are not foolproof. For example, AI models could inadvertently reconstruct sensitive information based on patterns learned during training. Furthermore, human oversight is often necessary to ensure that no privacy risks go undetected. As AI technologies evolve, continuous improvement and adaptation of these security protocols will be required.
"It is crucial that we address both the technical and ethical aspects of AI security to ensure a safe and responsible use of generative models." – AI Ethics Review Committee
Summary of Best Practices for Securing Sensitive Information
Strategy | Description |
---|---|
Data Masking | Eliminates sensitive identifiers in data before processing by the AI model. |
Output Filtering | Monitors and filters AI-generated content to detect and remove private information. |
Ethical Review | Ensures human oversight and the ethical implications of using AI-generated content. |
Best Practices for Safeguarding Personal Information in AI Systems
With the increasing use of AI across various industries, ensuring that personal data remains secure has become a critical concern. AI systems often require vast amounts of data to function effectively, making them potential targets for data breaches or misuse. Protecting this data not only ensures compliance with privacy laws but also maintains user trust in the technology. Implementing robust privacy measures is essential for AI developers and organizations using AI systems.
Adopting best practices for data protection is vital in preventing unauthorized access, misuse, or leakage of sensitive personal information. Below are key practices to follow when managing personal data within AI environments:
1. Data Minimization
One of the most effective methods for reducing privacy risks is minimizing the amount of personal data collected and processed by AI systems. This includes collecting only the data necessary for the task at hand.
- Limit the scope of data collection to specific purposes.
- Implement techniques like differential privacy or synthetic data generation to protect individual identities.
2. Data Encryption and Anonymization
Encrypting personal data both at rest and in transit is essential for protecting it from unauthorized access. Additionally, anonymizing data can significantly reduce privacy risks by ensuring that sensitive information is not linked to specific individuals.
- Apply encryption protocols such as AES for stored data.
- Use secure transfer protocols like TLS for data in transit.
- Anonymize datasets when possible, to prevent identification of individuals.
"Data anonymization helps in preserving privacy while allowing AI systems to operate effectively without compromising individual identities."
3. Transparent Data Handling and Consent
Transparency is key to ensuring that users understand how their data is being used. AI systems should be designed to provide clear explanations of data collection practices and obtain informed consent from users before their data is processed.
Best Practice | Description |
---|---|
Informed Consent | Ensure users are fully aware of the data collection, processing, and storage practices before agreeing to share their data. |
Data Access Transparency | Allow users to view and control their data, including how it is used and shared by AI systems. |
Addressing Bias and Discrimination in AI Models from a Privacy Perspective
As artificial intelligence systems continue to evolve, concerns about their ethical implications, particularly regarding bias and discrimination, have become increasingly prominent. These biases often emerge during the training phase of AI models, where data may reflect historical inequalities or social prejudices. From a privacy standpoint, the incorporation of such biases can amplify existing disparities, leading to discriminatory practices that affect individuals in various contexts, including healthcare, hiring, and law enforcement.
Addressing these biases requires not only technical solutions but also a comprehensive understanding of privacy concerns. Personal data used for training AI models must be protected to prevent misuse or the exacerbation of harmful stereotypes. This means adopting strategies that prioritize both fairness and privacy, ensuring that AI models serve all individuals equitably while safeguarding their personal information.
Key Considerations for Mitigating Bias in AI Models
- Data Selection and Representation: Ensuring that training datasets are diverse and inclusive to avoid reflecting existing societal biases.
- Privacy-Preserving Techniques: Implementing methods such as differential privacy to minimize the risk of revealing sensitive information during the model's training process.
- Transparency and Accountability: Developing clear protocols for auditing and monitoring AI models to detect and address potential biases regularly.
"The challenge is not only in detecting bias, but in ensuring that solutions do not compromise privacy or security." – AI Ethics Researcher
Steps to Enhance Fairness in AI without Compromising Privacy
- Data Anonymization: Stripping personal identifiers from datasets while retaining the information necessary to train fair models.
- Bias Auditing: Conducting regular reviews of AI models to assess the impact of biases on different demographic groups.
- Engagement with Affected Communities: Involving diverse groups of people in the development and review process to ensure broader representation and fairness.
Impact of Privacy-Protecting Measures on Bias Reduction
Privacy Measure | Impact on Bias Reduction |
---|---|
Data Masking | Prevents personal identifiers from influencing model outcomes, reducing the likelihood of biased decision-making based on demographic factors. |
Federated Learning | Allows models to be trained on decentralized data, preserving privacy while ensuring fairness through cross-site model updates. |
Differential Privacy | Ensures individual data points remain confidential, mitigating risks of discrimination based on overly specific personal information. |
Privacy-Preserving Techniques in Generative AI: Encryption and Differential Privacy
Generative AI models, especially those applied to sensitive data, raise concerns about user privacy and data protection. To mitigate these risks, privacy-preserving methods are essential in ensuring that the generated outputs do not inadvertently reveal private information. Among these, encryption and differential privacy stand out as crucial approaches to securing data while maintaining the functionality of the models.
Encryption techniques aim to safeguard the data during its processing, making it unreadable to unauthorized parties. Meanwhile, differential privacy introduces noise to the data, ensuring that individual data points cannot be traced back to specific users. Both approaches help to prevent the exposure of sensitive information while still enabling the benefits of generative AI systems.
Encryption Techniques
Encryption serves as a fundamental tool in protecting user data, especially when AI models process sensitive information. By transforming data into an unreadable format using a key, encryption ensures that only authorized parties can access the original content.
- Homomorphic Encryption: Allows operations on encrypted data without decrypting it, enabling privacy-preserving computations directly on sensitive data.
- Secure Multi-party Computation (SMC): Divides the computation process into smaller parts, which are independently processed, ensuring no party sees the full data.
- End-to-End Encryption: Protects data from the moment it is captured until it reaches its destination, ensuring privacy during data transmission.
Differential Privacy
Differential privacy ensures that the output of a generative AI system does not reveal information about any individual user, even if the attacker has access to the model's outputs. This is achieved by adding random noise to the data, making it impossible to identify specific entries in the dataset.
- Global Differential Privacy: Introduces noise globally to datasets before they are used for model training, ensuring privacy for all users.
- Local Differential Privacy: Perturbs data at the user level, protecting individual user privacy before sending data to the server.
- Query-based Differential Privacy: Limits the amount of information that can be inferred from queries made on the model by adding controlled noise.
Comparison of Techniques
Technique | Description | Use Case |
---|---|---|
Homomorphic Encryption | Allows computations on encrypted data without decryption | Secure data processing in cloud computing |
Secure Multi-party Computation | Splits computations across multiple parties, ensuring no party has access to the full data | Collaborative AI model training |
Differential Privacy | Adds noise to prevent identification of individual data points | Private data analysis in AI applications |
"Privacy-preserving methods like encryption and differential privacy are pivotal in ensuring that AI technologies do not compromise individual privacy while delivering valuable insights and innovations."