Generative Ai A16z
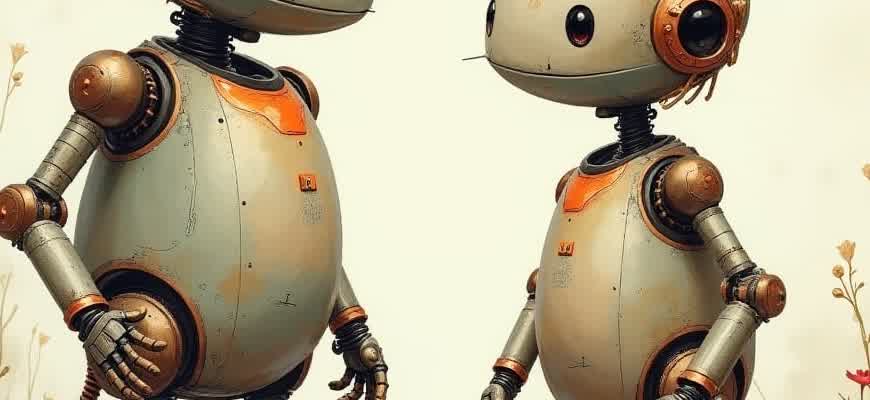
The landscape of artificial intelligence is evolving rapidly, with new advancements pushing the boundaries of what's possible. Among the key players in this transformation is Andreessen Horowitz (A16z), a prominent venture capital firm that has been at the forefront of supporting generative AI startups. A16z's deep involvement in the AI ecosystem highlights their commitment to exploring the potential of these technologies to revolutionize industries.
Generative AI refers to the subset of AI systems that are designed to create new content–whether it's images, text, music, or code. These models are trained on vast datasets and leverage deep learning techniques to produce novel outputs. With applications ranging from creative industries to software development, generative AI is transforming how businesses operate and interact with customers.
"Generative AI holds the potential to redefine creative processes and unlock entirely new ways of problem-solving." - A16z Report on AI Trends
In recent years, A16z has made significant investments in AI companies that are pushing the envelope in this space. Below are some of the key areas where generative AI is making an impact:
- Content creation: Automated writing, design, and music production.
- Software development: AI-driven code generation and debugging tools.
- Healthcare: AI models for drug discovery and diagnostic assistance.
The firm has also created several partnerships with AI startups, aiming to accelerate the adoption of generative technologies across various sectors. These investments not only provide capital but also shape the future trajectory of generative AI innovation.
Key Areas of Generative AI | Impact |
---|---|
Content Creation | Automates creative workflows and enhances productivity. |
Software Development | Improves efficiency through code automation and problem-solving tools. |
Healthcare | Speeds up research and clinical applications with AI-driven analysis. |
How A16z's Generative AI Can Drive Business Transformation
Generative AI, backed by A16z's cutting-edge investments, offers businesses unparalleled opportunities to innovate and scale operations. By integrating AI models that learn from data and generate new content, companies can automate key processes, personalize customer experiences, and reduce operational costs. This technology empowers businesses to create more engaging products, streamline workflows, and enhance decision-making in ways that were previously impossible.
The real power of A16z's approach to generative AI lies in its adaptability across various industries, from customer service to product development. As businesses look to optimize efficiency and increase market competitiveness, generative AI becomes an essential tool in transforming day-to-day operations and strategic initiatives.
Key Benefits of Generative AI for Business
- Personalization at Scale: AI models can tailor content and recommendations for customers, driving deeper engagement and satisfaction.
- Improved Productivity: Automate repetitive tasks and processes, allowing teams to focus on more strategic, high-value work.
- Cost Reduction: AI can optimize resource allocation and reduce the need for manual labor, lowering operational costs.
- Faster Innovation: With generative AI, businesses can prototype new products or services more rapidly, accelerating time to market.
How A16z’s Approach Supports Business Transformation
With A16z's guidance, businesses gain access to advanced AI solutions that are tailored to their specific needs, enabling a quicker and more efficient transition to AI-powered operations. This includes:
- Scalable AI Models: A16z helps integrate AI models that evolve with your business, growing alongside changing demands.
- Advanced Data Insights: Leverage AI to analyze vast amounts of data for better decision-making and trend forecasting.
- Ethical AI Implementation: A16z emphasizes ethical considerations, ensuring AI adoption aligns with company values and societal standards.
Generative AI from A16z is not just a technological upgrade–it's a strategic asset that drives long-term business growth and resilience.
Real-World Use Cases
Industry | AI Application | Business Impact |
---|---|---|
Retail | AI-driven personalized shopping experiences | Increased customer loyalty and higher conversion rates |
Finance | AI for fraud detection and risk assessment | Reduced fraud risk and enhanced compliance |
Healthcare | Generative AI for medical imaging and diagnostics | Faster, more accurate diagnoses, leading to improved patient outcomes |
Understanding Generative AI: Key Concepts and Applications
Generative AI represents a subset of artificial intelligence that focuses on creating new data, content, or solutions rather than simply analyzing or processing existing data. By leveraging advanced machine learning models, generative algorithms are capable of producing novel outputs, including text, images, music, and even code. These systems are trained on large datasets and learn the patterns, structures, and relationships within the data, allowing them to generate realistic and coherent results based on the input they receive.
This technology has gained significant attention due to its wide range of applications, from enhancing creative processes to streamlining business operations. While generative AI has the potential to revolutionize various industries, understanding the core principles behind its operation is crucial for maximizing its value and ensuring ethical and responsible use.
Core Concepts of Generative AI
- Generative Models: These models are trained to create new data based on learned patterns. Examples include Generative Adversarial Networks (GANs) and Variational Autoencoders (VAEs).
- Training Data: Generative AI systems learn from vast amounts of data to identify patterns and structures that they can later use to generate new content.
- Optimization: The process of adjusting the model’s parameters to improve its ability to produce realistic and high-quality outputs.
- Latent Space: A mathematical representation of the data that enables the model to generate new variations by exploring different points in this space.
Applications of Generative AI
- Content Creation: Generative AI is widely used in generating text, images, and videos for media, advertising, and entertainment. Models like GPT-3 and DALL·E have revolutionized content production.
- Healthcare: In drug discovery, generative models can create new chemical compounds with specific properties, potentially speeding up the development of treatments.
- Design: Designers use generative algorithms to create innovative product designs, architecture, and even fashion, allowing for the exploration of novel concepts.
- Gaming: Generative AI aids in creating dynamic game environments, characters, and stories that adapt to user input, enhancing player experience.
Key Challenges and Ethical Considerations
"While generative AI brings transformative potential, it also raises significant ethical concerns, including the creation of misleading content and the misuse of AI-generated materials."
Challenge | Potential Impact |
---|---|
Data Privacy | Generative AI models may inadvertently expose sensitive information by recreating or synthesizing data that is too similar to private data. |
Deepfake Technology | The ability to create hyper-realistic fake content can lead to misinformation, fraud, and manipulation. |
Bias and Fairness | Generative AI models may perpetuate existing biases present in the training data, leading to unfair or discriminatory outputs. |
How A16z’s Generative AI Transforms Marketing Content Creation
In the evolving landscape of digital marketing, A16z's generative AI solutions are providing a groundbreaking shift in how businesses approach content creation. Leveraging machine learning, these tools enable marketers to streamline the generation of high-quality, tailored content with minimal human input. This reduces both time and cost, while enhancing personalization and engagement at scale. With sophisticated natural language processing capabilities, the AI can understand context, tone, and audience needs, ensuring content resonates on a deeper level.
One of the key advantages of A16z’s AI technology is its ability to analyze vast amounts of consumer data and generate targeted content that speaks directly to the interests of specific demographics. By integrating advanced algorithms, it can create text, graphics, and even videos that align with a brand’s voice, optimizing for maximum impact across multiple channels. The result is more effective campaigns and higher conversion rates.
Key Features of A16z’s Generative AI for Marketing
- Content Personalization: AI generates tailored messages that resonate with specific audiences based on data analysis.
- Efficiency: Significantly reduces content creation time, allowing teams to focus on strategic activities.
- Scalability: AI can produce high volumes of content across various formats without compromising quality.
- Enhanced Engagement: Through AI-driven insights, content is more likely to engage and convert potential customers.
Examples of AI-Driven Content Creation
- Social Media Posts: Quickly generate personalized, attention-grabbing posts for various platforms.
- Email Campaigns: Create customized email copy that speaks directly to the needs and desires of the target audience.
- Ad Copy: Generate high-converting ad copy that adapts to different market segments and campaign objectives.
Impact on Marketing Teams
Aspect | Before A16z AI | After A16z AI |
---|---|---|
Time Spent on Content Creation | Hours or days per piece | Minutes per piece |
Content Customization | Limited and generic | Highly personalized |
Engagement Rate | Varies, often low | Increased significantly |
"A16z's generative AI has revolutionized the content creation process by automating the tedious aspects of marketing, allowing teams to focus on strategy and creative direction."
Enhancing User Engagement with AI-Powered Custom Experiences
With the rise of artificial intelligence, brands are now able to offer deeply personalized interactions with their customers. By leveraging advanced AI models, businesses can create tailored experiences that speak directly to individual preferences, significantly improving user engagement. AI-generated content not only provides a more dynamic interaction but also enhances customer satisfaction by responding to needs in real-time.
By integrating AI systems into customer-facing platforms, companies can craft unique pathways that keep users involved for longer periods. These systems learn continuously from user behavior and feedback, enabling the creation of highly relevant suggestions, content, and offers that resonate on a personal level.
Key Strategies for AI-Driven Personalization
- Data Collection & Analysis: Use AI to analyze user behavior and preferences through data points such as browsing history, clicks, and engagement patterns.
- Dynamic Content Generation: AI can generate content that adapts to the user's interests, such as product recommendations, articles, and offers.
- Real-time Customization: Personalized messaging can be adjusted based on live interactions, keeping the experience relevant and timely.
- Predictive Analytics: AI can predict customer needs based on historical data and trends, providing proactive solutions before the user even realizes a need.
Impact on Engagement Metrics
Metric | Before AI | After AI |
---|---|---|
Customer Retention Rate | 60% | 85% |
Average Session Duration | 3 minutes | 7 minutes |
Conversion Rate | 2% | 5% |
"AI's ability to continually optimize the customer journey through personalized experiences has transformed traditional engagement strategies. It's no longer about pushing generic content, but delivering exactly what each individual needs in real time."
Cost Efficiency in Product Design and Development with Generative AI
Generative AI has significantly impacted the design and development sectors by streamlining workflows and reducing operational costs. With advancements in AI technology, companies are able to automate previously time-intensive tasks, thereby accelerating production cycles and minimizing the need for expensive manual labor. As a result, businesses can achieve more with fewer resources, all while maintaining high levels of creativity and innovation.
From concept creation to the prototyping phase, AI tools are being integrated into every step of the design process. By leveraging AI's ability to generate and optimize designs based on user input and existing data, companies can experiment with a vast range of options without incurring high costs. In this way, generative models help reduce the number of revisions and iterations typically needed during the development phase.
Key Areas Where AI Reduces Costs
- Automated Concept Generation: AI can quickly generate a wide variety of design concepts based on predefined parameters, minimizing the need for manual ideation.
- Material Optimization: Using AI models, designers can analyze material costs and optimize choices to minimize waste and reduce overall expenses.
- Rapid Prototyping: AI-driven software enables faster, more efficient prototyping processes, reducing both time and resource allocation.
- Iterative Testing: AI tools can simulate performance and user feedback, helping teams identify and fix issues before physical prototypes are created, saving both time and money.
Examples of Cost Reduction through Generative AI
- Product Design: AI can analyze customer feedback and generate product design options that meet specific market needs, decreasing design time and increasing alignment with customer preferences.
- Automated Coding for Software Development: Generative AI can write portions of code, reducing the development time needed for building new software applications.
- Supply Chain Optimization: AI-powered predictive models help companies optimize inventory and distribution strategies, reducing overhead and unnecessary stockpiling.
"Generative AI is transforming the product development lifecycle, making it more efficient and cost-effective, while also opening up new creative possibilities for designers and engineers." – A16z Insights
Cost Reduction in Practice: A Comparative Table
Traditional Approach | Generative AI Approach | Cost Reduction |
---|---|---|
Manual design iteration | AI-generated design suggestions | 50% reduction in design time |
Physical prototyping | AI-driven simulations | 40% reduction in prototyping costs |
Extensive manual testing | AI-enhanced testing and optimization | 30% reduction in development costs |
Enhancing Data-Driven Decisions with Generative AI Tools
Generative AI tools are transforming the way organizations approach data-driven decision-making. These advanced technologies can help businesses by providing insights that go beyond traditional data analysis methods. By leveraging machine learning and artificial intelligence, generative models create predictive models, simulate outcomes, and enhance the decision-making process by analyzing vast amounts of structured and unstructured data in real-time.
The power of these tools lies in their ability to generate new, meaningful data points from existing datasets, enabling decision-makers to explore a broader range of possibilities. With improved predictive capabilities, generative AI can identify trends, uncover hidden patterns, and propose optimized strategies tailored to specific goals. These tools not only increase the accuracy of decisions but also help mitigate risks associated with uncertainty and biases.
Key Benefits of Generative AI in Data-Driven Decision Making
- Advanced Data Synthesis: By generating synthetic data, AI tools can fill gaps in incomplete datasets, enhancing the accuracy of predictive models.
- Scenario Simulation: Generative AI can simulate various business scenarios, allowing decision-makers to evaluate potential outcomes before committing to a course of action.
- Real-Time Decision Support: With AI analyzing data continuously, organizations can make adjustments to strategies almost instantaneously based on emerging trends.
Practical Applications
- Customer Insights: AI can generate customer behavior predictions, enabling businesses to tailor marketing campaigns and optimize sales strategies.
- Supply Chain Optimization: Generative models simulate supply chain disruptions and suggest the most efficient responses to minimize delays and costs.
- Financial Forecasting: AI tools analyze financial data to generate accurate forecasts, helping businesses plan for future market conditions.
Impact on Business Strategy
"Generative AI enables companies to make smarter decisions by providing a deeper understanding of both historical data and future trends. This shift from reactive to proactive decision-making is a game-changer in competitive industries."
Data-Driven Decision Framework
Step | Description |
---|---|
Data Collection | Gather structured and unstructured data from various sources, such as customer interactions and market trends. |
Data Processing | Use generative AI to clean, filter, and synthesize the data, preparing it for analysis. |
Analysis & Simulation | AI generates multiple predictive models to simulate different business scenarios and potential outcomes. |
Decision Making | Utilize AI-generated insights to guide strategic decisions and actions. |
Integrating A16z's Generative AI into Your Existing Technology Ecosystem
Integrating A16z's generative AI technologies into your existing infrastructure can significantly enhance the capabilities of your systems. The process involves careful planning to ensure smooth integration with minimal disruptions to ongoing operations. To achieve this, companies must consider factors such as compatibility with existing tools, scalability, and the specific requirements of their workflows. Below are some essential steps for a seamless integration.
One of the first steps is evaluating how generative AI aligns with your business goals. The technology can be deployed across various functions, such as content generation, data analysis, and predictive modeling. Once this alignment is established, integration efforts can be structured around the existing systems, whether they're cloud-based platforms or on-premise solutions.
Key Integration Steps
- Assessment of Compatibility: Ensure the generative AI tools are compatible with your current tech stack, whether it involves APIs, cloud services, or data pipelines.
- Infrastructure Evaluation: Analyze the computational resources needed for AI models and assess whether your existing infrastructure can support these demands.
- Scalability Considerations: Plan for future scalability to handle larger datasets or more complex models without significant rework.
- Data Integration: Integrate AI solutions with your data pipelines to allow smooth flow and access to the information needed for training and analysis.
Best Practices
- Automate Workflows: Streamline processes by integrating AI-driven decision-making directly into business workflows.
- Real-time Monitoring: Implement monitoring systems to track AI performance and ensure its output aligns with expected results.
- Continuous Learning: Set up mechanisms for AI models to continuously learn from new data, ensuring their outputs remain relevant.
Integrating A16z's generative AI offers a competitive advantage, but it requires thoughtful planning and ongoing optimization for long-term success.
Sample Integration Table
Step | Action | Tools/Resources |
---|---|---|
Compatibility Check | Ensure AI tools work with your APIs, databases, and services | API Documentation, System Logs |
Infrastructure Scaling | Evaluate hardware or cloud scaling requirements | Cloud Platforms (AWS, GCP), On-prem Servers |
Data Integration | Integrate AI with existing data pipelines | ETL Tools, Data Warehouses |
Measuring ROI: How to Track Success with Generative AI A16z
When adopting Generative AI, understanding its impact on business performance is critical. Measuring the return on investment (ROI) can be challenging due to the complexity of AI systems and the different metrics involved. To track the success of these AI implementations, businesses need to focus on key performance indicators (KPIs) that directly reflect the value generated. It's essential to evaluate both quantitative and qualitative outcomes to paint a clear picture of AI's impact on the organization.
Key factors to measure ROI include cost savings, productivity improvements, and revenue generation. Monitoring these indicators can help businesses determine how effectively Generative AI is delivering value and where adjustments might be necessary. A structured approach will ensure that companies can justify their investment and make informed decisions about scaling AI solutions.
Key Metrics for Measuring Success
- Cost Reduction: Analyze how AI minimizes operational costs by automating tasks that previously required human intervention.
- Increased Efficiency: Track how AI streamlines processes, reduces time spent on repetitive tasks, and enhances overall productivity.
- Revenue Growth: Evaluate the impact of AI on generating new revenue streams or enhancing existing ones through improved customer engagement and product innovation.
Steps for Tracking AI ROI
- Define Clear Objectives: Before implementing AI, set clear and measurable goals that align with business objectives.
- Track Baseline Metrics: Establish a baseline for current performance in relevant areas, such as cost, productivity, and revenue.
- Monitor Post-Implementation Results: Continuously monitor the performance of AI systems to track improvements and identify areas of concern.
- Analyze Data: Use analytics tools to compare pre- and post-implementation results, identifying trends and areas of significant impact.
- Refine and Optimize: Use insights gained to refine AI models, optimizing them for even greater returns.
Example of ROI Metrics
Metric | Pre-AI | Post-AI | Change (%) |
---|---|---|---|
Operational Cost | $500,000 | $350,000 | -30% |
Productivity (Tasks per Day) | 100 | 200 | +100% |
Revenue Growth | $1,000,000 | $1,200,000 | +20% |
"To truly assess the ROI of Generative AI, businesses must move beyond simple cost savings and consider the broader impact on innovation and growth." – A16z