Generative Ai Cnbc
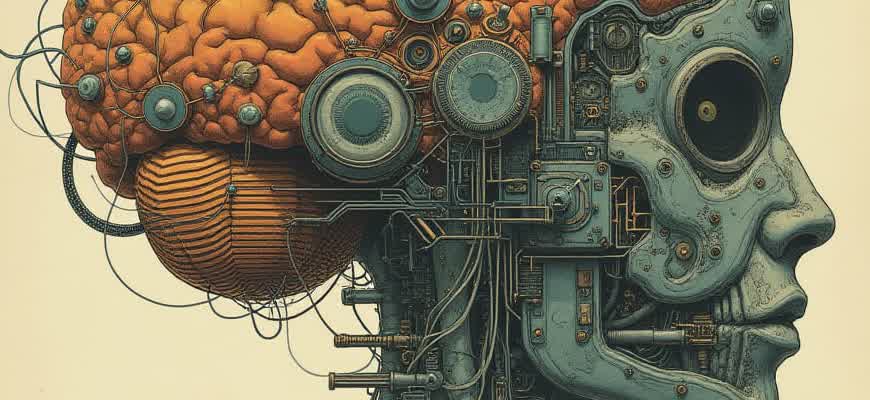
Artificial intelligence systems capable of producing text, images, and code are redefining business media narratives. CNBC, a major player in financial journalism, actively tracks how such technologies disrupt corporate operations, workforce structures, and investor expectations. Their reporting often focuses on real-world applications and market reactions.
- Corporate adoption of synthetic content tools
- Regulatory debates on machine-generated media
- Stock market responses to AI announcements
"Major enterprises are not just experimenting–they're reallocating entire budgets to machine-driven content creation," CNBC reported in a March 2025 segment.
Key areas of CNBC’s AI reporting include:
- Quarterly earnings impacts tied to AI implementation
- Shifts in advertising and media production strategies
- Ethical concerns around automated journalism
Company | AI Integration | CNBC Report Date |
---|---|---|
Adobe | Image synthesis and automated design | Feb 14, 2025 |
Meta | AI-generated avatars and ad copy | Mar 3, 2025 |
How to Promote the CNBC-Branded Generative AI Offering
To effectively market CNBC’s advanced AI solution tailored for financial content, the focus should be on demonstrating real-world use cases and measurable outcomes. Instead of broad claims, showcase how the product helps traders, analysts, and business journalists accelerate decision-making and boost productivity.
Strategic promotion across digital and broadcast platforms must emphasize trust, data accuracy, and seamless integration with CNBC's financial news infrastructure. The message should highlight how this tool transforms raw data into actionable insights with minimal effort.
Promotion Strategies
“Speed to insight is no longer optional – it’s the baseline expectation.”
- Launch exclusive AI-driven market insight reports weekly on CNBC.com to build credibility.
- Feature testimonials from institutional investors and newsroom editors using the tool daily.
- Develop short explainer segments aired on CNBC TV during market hours, showing real-time use.
- Host live demos and webinars in partnership with financial influencers and analysts.
- Offer limited-time enterprise trials to fintech firms and hedge funds with tailored onboarding.
- Integrate the tool with CNBC Pro subscriptions for added value and upsell opportunities.
Promotion Channel | Target Audience | Expected Outcome |
---|---|---|
CNBC TV Segments | Retail and Institutional Investors | Boost product awareness |
Web-Based Demo Campaigns | Finance Professionals | Lead generation and trial sign-ups |
Email Outreach to Pro Users | Existing Subscribers | Increase upgrade conversions |
Identifying High-Intent Use Cases for Generative AI in Financial Media
In financial newsrooms, AI-driven tools are rapidly moving from experimental to essential, especially in scenarios where the user's goal is transaction-oriented or decision-driven. Pinpointing these high-intent contexts allows financial media outlets to prioritize AI deployment where it adds the most value–delivering personalized, data-rich insights that support investor actions, portfolio decisions, or strategic planning.
By examining user behavior and content demand, platforms can uncover moments where intent is clearest–such as real-time market analysis, earnings coverage, or forecast-driven stock exploration. In these cases, generative systems must synthesize large datasets, surface relevant metrics, and deliver insight with zero latency.
Key Applications with Clear User Intent
- Earnings Calls Summarization: Automatically converting transcripts into analyst-style takeaways for retail investors.
- Market Movers Analysis: Explaining abnormal stock movements using real-time sentiment, volume, and macroeconomic data.
- Portfolio Impact Simulation: AI-driven forecasting tools showing how events affect user-saved assets or watchlists.
Insight: Engagement spikes significantly when AI-generated content answers a user's implicit question: "What does this mean for me?"
- Track behavioral signals (e.g., watchlist edits, search terms) to infer intent level.
- Apply generative models only where user actions suggest near-term decision-making.
- Integrate tools directly into user workflows like portfolio dashboards or trade alerts.
Use Case | Trigger Event | AI Response |
---|---|---|
Rate Hike Forecast | Fed announcement scheduled | Customized scenario modeling |
Stock Volatility Surge | Unusual trading volume | Contextual news generation with peer comparison |
IPO Watch | User follows IPO ticker | AI-curated pros/cons summary |
Creating Targeted Messaging That Aligns with CNBC’s Core Audience
Rather than broad narratives, content should focus on actionable insights, market relevance, and forward-looking perspectives. This includes integrating data-driven storytelling, highlighting key financial movements, and offering nuanced viewpoints that reflect a sophisticated understanding of global economic trends.
Essential Elements for Audience-Centric Messaging
Strong messaging for CNBC’s audience should prioritize accuracy, financial relevance, and a tone of professional authority – without sacrificing clarity.
- Precision over generality: Avoid vague statements; use clear financial terminology aligned with investment and market contexts.
- Time sensitivity: Messages should reflect current economic cycles, regulatory developments, and global business shifts.
- Expert tone: Adopt a confident, analytical voice that mirrors CNBC’s editorial standards.
- Reference performance metrics, economic indicators, and market sentiment data.
- Align messaging with investor psychology – risk, return, and opportunity.
- Use segmentation logic to address varied interests (e.g., retail traders vs. institutional analysts).
Audience Segment | Message Focus | Content Format |
---|---|---|
Institutional Investors | Macroeconomic trends, regulatory shifts | Deep-dive reports, infographics |
Retail Traders | Technical analysis, trading signals | Short-form videos, newsletters |
Financial Advisors | Portfolio strategies, client sentiment | Webinars, expert interviews |
Building Trust Through Data-Driven AI Demonstrations
Transparent AI performance metrics are critical in convincing stakeholders of the reliability and fairness of generative models. By showcasing reproducible test outcomes across varied datasets, companies can demystify how these systems operate under real-world conditions.
When organizations present empirical benchmarks–such as accuracy, latency, and error rates–decision-makers gain confidence in the system’s integrity. Rather than abstract claims, data-backed demonstrations offer quantifiable proof of capability and limitations.
Core Strategies for Gaining Confidence in AI
- Utilize controlled scenario testing to show how the model behaves in specific use cases.
- Compare output quality against human benchmarks for measurable evaluation.
- Make evaluation datasets publicly accessible for third-party verification.
“AI systems should be judged by their output, not their claims. Demonstrated consistency builds trust faster than any promise.” – Enterprise AI Lead, TechForum 2025
- Document and share model versioning with performance deltas.
- Include bias detection metrics to address ethical concerns directly.
- Offer live walkthroughs of AI decision logic for auditability.
Metric | Test Result | Human Baseline |
---|---|---|
Text Summarization Accuracy | 92.1% | 88.3% |
Image Captioning Precision | 87.6% | 85.0% |
Latency (avg. response) | 210ms | N/A |
Using CNBC's Authority to Stand Apart in the AI Landscape
This strategic integration enables CNBC to create a unique AI assistant experience tailored for professionals and investors. Rather than generic outputs, users get intelligent narratives, market alerts, and financial interpretations with traceable sources – a critical advantage in decision-heavy environments.
Key Differentiators Anchored in CNBC's Legacy
- Credibility: AI responses grounded in CNBC’s editorial standards provide verified financial intelligence.
- Contextual Accuracy: Responses are enriched by CNBC’s archives and real-time reporting.
- Market Relevance: User prompts are interpreted with an understanding of financial cycles, policy changes, and investor behavior.
CNBC's AI assistant doesn't just predict trends – it explains them, backed by named sources and current financial narratives.
- Pull data directly from CNBC’s proprietary economic indicators and live market feeds.
- Surface analyst commentary and CEO interviews in response to user questions.
- Translate breaking news into investment insights, tailored to user portfolios.
Feature | Generic AI | CNBC-Integrated AI |
---|---|---|
Market Context | Basic economic terms | Live market correlations with editorial commentary |
Trust Level | Unverified open data | Sourced from CNBC reporters and analysts |
Audience Relevance | General users | Investors, analysts, finance professionals |
Optimizing Landing Pages for AI-Driven User Engagement
As machine learning algorithms become more adept at personalizing digital experiences, landing pages must evolve to meet new standards of relevance and immediacy. By integrating adaptive components that respond to inferred user intent, businesses can drive higher conversion rates and improve overall interaction quality.
Leveraging predictive analytics and behavior modeling, AI systems can dynamically alter content blocks, CTAs, and product placements based on visitor profiles. This transforms static pages into intelligent entry points that resonate with individual users in real time.
Key Techniques for Intelligent Landing Page Enhancement
- Content Modularization: Use AI to serve personalized messaging modules tailored to user segments.
- Real-Time UX Adjustments: Alter page layout or CTA prominence based on scroll depth, click behavior, or referral source.
- Predictive CTA Placement: Position interactive elements where user attention is forecasted to peak.
AI-optimized landing pages have shown up to a 30% increase in engagement metrics when personalization is applied to above-the-fold content.
- Train AI models with interaction data from past campaigns.
- Define key engagement indicators (e.g., time on page, scroll rate).
- Deploy A/B testing for AI-generated variants to refine algorithms.
AI Element | Impact on Engagement | Use Case |
---|---|---|
Behavior-Based Recommendations | +25% click-through rate | Dynamic product highlights |
Context-Aware Headlines | +18% average session duration | Geo-based messaging |
Intent-Driven Forms | +40% form completion | Shortened input fields based on user history |
Creating Business Impact Through Practical Case Studies
Developing case studies that effectively illustrate the tangible business benefits of generative AI involves a clear understanding of both the technology and its application. By focusing on specific challenges and solutions, companies can showcase the real-world impact of AI systems in various sectors. These case studies are powerful tools for demonstrating the value of generative AI beyond theoretical applications, presenting actionable insights and measurable results.
When creating such case studies, it is essential to structure them in a way that highlights key business outcomes, scalability, and potential for innovation. This allows stakeholders to grasp the specific advantages generative AI brings to their industry or field.
Key Components of Effective Case Studies
- Problem Definition: Clearly describe the business challenge that the AI solution is addressing.
- Solution Implementation: Detail how generative AI technologies were applied, including methodologies and tools used.
- Business Impact: Showcase measurable results, such as cost reduction, increased efficiency, or improved customer satisfaction.
- Lessons Learned: Provide insights on what worked, challenges faced, and areas for improvement.
"By focusing on real-world applications, generative AI can be positioned as a strategic tool that delivers value rather than just a theoretical concept."
Example Case Study Breakdown
Company | Problem | Solution | Results |
---|---|---|---|
Company A | Manual data processing inefficiencies | Automated data analysis using generative AI | 40% reduction in processing time |
Company B | Customer engagement lag | Personalized content generation with AI | 30% increase in customer retention |
"The success of these case studies lies in how they translate AI innovations into concrete, business-driven outcomes."
Designing Effective Lead Nurturing Strategies for AI-Inquisitive Audiences
When engaging prospects who are curious about generative AI, it’s crucial to tailor your communication strategy to align with their knowledge and interests. A targeted nurturing sequence helps gradually build trust and move them closer to conversion. Understanding the specific needs and pain points of these individuals can make a significant difference in the effectiveness of your outreach efforts.
By crafting content and interactions that are not overwhelming but rather informative, you can ensure that the journey from awareness to decision-making is smooth and personalized. Here’s a breakdown of key steps to design these lead nurture sequences:
Steps to Create an Effective Lead Nurturing Journey
- Segment your leads – Group prospects based on their familiarity with AI concepts, their role in the industry, or their specific interest in the technology.
- Build an educational path – Provide resources, such as white papers, articles, and case studies, that progressively introduce AI concepts.
- Introduce interactive content – Create opportunities for engagement through webinars, Q&A sessions, and demos to address specific questions and challenges.
- Use personalized email sequences – Tailor your email outreach to the specific stage of the prospect’s journey, from introductory to more technical information.
Key takeaway: A well-planned nurturing sequence is vital for guiding prospects through the stages of learning and decision-making in the AI field.
Important Elements in the Sequence
Content Type | Purpose |
---|---|
Introductory Articles | Educate about the basics of generative AI and its potential applications. |
Case Studies | Show practical implementations and real-world success stories to build credibility. |
Webinars | Engage and offer direct interaction for prospects to ask questions and gain deeper insights. |
Critical point: Structure the nurturing process to be adaptive, ensuring the content grows in complexity as the prospect progresses in their understanding.