Neural Network Voice
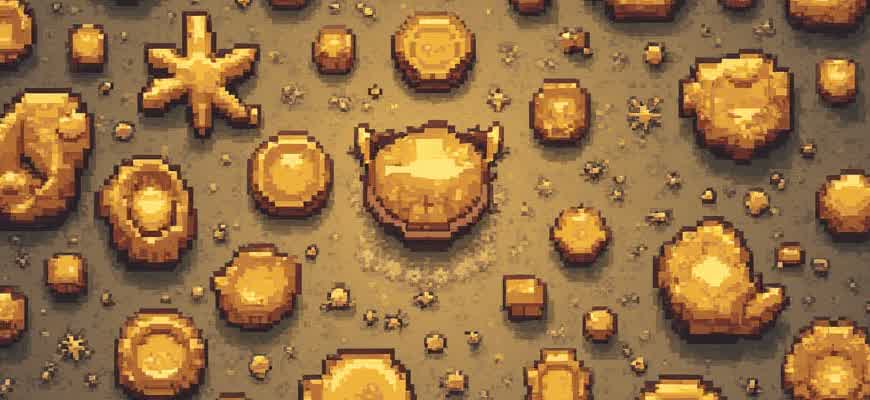
The integration of neural networks in voice technology has significantly advanced the way machines interpret and generate human speech. These systems utilize deep learning models to simulate the nuances of human vocal patterns, making interactions with devices more natural and intuitive. Below are key areas where neural networks play a pivotal role:
- Speech Recognition: Converting spoken language into text through deep learning models.
- Voice Synthesis: Generating human-like speech from text input, allowing more natural communication with devices.
- Emotion Detection: Analyzing tone and inflection to understand emotional context in speech.
To better understand how neural networks process voice data, let's look at some common steps involved:
- Data Collection: Accumulating vast amounts of voice samples to train the system.
- Model Training: Using deep neural networks to learn patterns in speech data.
- Real-time Processing: Applying the trained model to recognize or generate voice in real-time scenarios.
"Neural networks enable machines to understand and replicate the subtleties of human speech, enhancing user experience in voice-driven applications."
The following table outlines some of the key applications of neural network-based voice systems:
Application | Description |
---|---|
Virtual Assistants | Enable voice-based interaction, such as Apple's Siri or Amazon's Alexa. |
Speech-to-Text | Convert spoken language into written text, used in transcription and voice-controlled systems. |
Text-to-Speech | Generate human-like speech from text, improving accessibility and communication. |
How Neural Networks Enhance Voice Recognition for Companies
In recent years, businesses have increasingly adopted neural networks to optimize speech recognition processes, boosting efficiency and accuracy. These advanced systems use deep learning techniques to understand and transcribe spoken language with remarkable precision. By training on vast datasets, neural networks can recognize subtle nuances, accents, and variations in speech, making them indispensable for industries such as customer service, healthcare, and finance.
The integration of neural networks into voice recognition technologies enables companies to automate tasks, improve customer interactions, and gather valuable insights. These systems are continuously evolving, adapting to new languages, dialects, and terminology. As a result, they reduce manual transcription errors, improve data accuracy, and enhance the overall user experience.
Key Benefits of Neural Networks in Speech Recognition
- Improved Accuracy – Neural networks process audio data with exceptional precision, overcoming issues such as background noise, multiple speakers, and varying speech patterns.
- Real-time Transcription – Voice recognition powered by neural networks can transcribe conversations almost instantly, enabling quick responses in live settings.
- Language Adaptability – These systems learn from a wide range of dialects and terminologies, ensuring that businesses can tailor their speech recognition tools for global use.
- Cost Efficiency – By automating voice-based tasks, companies can reduce labor costs and allocate resources to more strategic functions.
Applications Across Industries
- Customer Service – Automates call center operations, providing quicker resolutions through AI-driven conversations.
- Healthcare – Assists in transcribing medical notes, enabling doctors to focus more on patient care.
- Financial Services – Improves voice commands for transactions and enhances security with voice biometrics.
"The integration of neural networks has revolutionized how businesses approach voice interaction, creating more responsive, reliable, and accurate systems that save time and resources."
Impact on Operational Efficiency
Before Neural Networks | After Neural Networks |
---|---|
Manual transcription processes prone to errors and delays. | Automated transcription with near-perfect accuracy in real-time. |
Limited language support, often requiring human intervention. | Multilingual support, learning new dialects and terminologies autonomously. |
Increased labor costs for voice-based tasks. | Cost reduction through automation of voice-related processes. |
Streamlining Customer Support with Neural Network-Driven Voice Assistance
Customer support is a critical function for businesses aiming to maintain customer satisfaction. With the rise of artificial intelligence and machine learning, neural network-driven voice assistants are reshaping how support services operate. These systems leverage deep learning models to understand, process, and respond to customer queries, automating tasks that were once handled solely by human agents.
By integrating neural networks into voice-based customer service systems, companies can achieve significant improvements in efficiency, response time, and scalability. The ability of these systems to comprehend natural language and adapt to various accents and speech patterns enhances the user experience, making interactions smoother and more intuitive.
Key Benefits of Neural Network-Driven Voice Assistance
- Cost Reduction: Reduces the need for a large support team by automating repetitive tasks.
- 24/7 Availability: Offers round-the-clock customer service without breaks or downtime.
- Faster Response Times: Delivers instant responses, improving customer satisfaction and reducing wait times.
- Personalized Interactions: Uses data from previous interactions to tailor responses, making each customer feel valued.
How Neural Network Voice Assistants Operate
- Speech Recognition: The neural network first transcribes the customer's spoken input into text.
- Intent Identification: It then determines the purpose of the query, such as troubleshooting or product inquiry.
- Contextual Understanding: The system interprets the context of the conversation, considering previous interactions and customer history.
- Response Generation: Using this understanding, the neural network generates an appropriate response, often with natural-sounding speech output.
Neural network-driven voice assistants provide a scalable solution that not only optimizes customer support but also reduces human error and operational costs.
Comparison of Traditional vs. Neural Network-Driven Support Systems
Aspect | Traditional Support | Neural Network-Driven Support |
---|---|---|
Response Time | Longer, as human agents process requests | Instant, automated responses |
Scalability | Limited by available human agents | Highly scalable, can handle thousands of requests simultaneously |
Cost Efficiency | Higher, due to labor costs | Lower, as most interactions are automated |
Enhancing Accessibility with AI-Powered Voice Solutions
Artificial intelligence is revolutionizing the way individuals with disabilities interact with technology, particularly through advanced voice recognition systems. These solutions not only assist in performing everyday tasks but also open up new possibilities for greater independence. As voice technologies become more accurate and context-aware, they are bridging the gap between accessibility barriers and digital interaction.
AI-driven voice systems are proving to be invaluable tools in enhancing the lives of those with visual impairments, mobility challenges, and other disabilities. By enabling voice control, these systems offer users the ability to interact with devices, access information, and navigate online spaces more effectively. The integration of neural network-based speech recognition has further improved the overall experience, providing more natural and seamless interactions.
Key Benefits of Voice Solutions for Accessibility
- Enhanced Communication: AI-powered voice assistants enable individuals with speech impairments to communicate more easily by transcribing spoken words into text or converting text into spoken language.
- Independent Navigation: Voice commands can guide users through physical and digital environments, allowing them to access websites, operate smart devices, and move through unfamiliar spaces without assistance.
- Personalization: AI systems adapt to individual users' speech patterns, preferences, and needs, providing a customized experience tailored to each person's requirements.
Examples of AI-Powered Voice Applications
- Speech-to-text apps for individuals with hearing impairments.
- Voice-controlled home automation for people with mobility limitations.
- Smart assistants designed to help people with visual impairments navigate digital content.
"AI voice solutions are no longer just convenience tools. They are critical aids that empower users with disabilities to participate more fully in both digital and physical environments."
Impact on Daily Life
By integrating these voice-driven technologies, users experience a profound shift in how they approach daily tasks. Accessibility features powered by AI not only enhance productivity but also foster a sense of inclusion. For example, voice-controlled applications are helping people with disabilities perform tasks such as sending emails, making calls, and controlling their environment through smart home devices.
Feature | Impact |
---|---|
Speech Recognition | Enables individuals with physical disabilities to control devices and interact with digital content hands-free. |
Natural Language Processing | Improves the accuracy of voice interactions, making the technology more intuitive and accessible for users with varied speech patterns. |
Real-time Transcription | Assists people with hearing impairments by converting spoken language into readable text. |
Reducing Operational Costs with AI-Driven Voice Solutions for Customer Support
With the rise of artificial intelligence and machine learning, call centers have begun integrating automated voice systems to handle routine customer interactions. These AI-driven solutions are able to handle large volumes of calls, decreasing the need for human agents in basic queries, and significantly reducing operational costs. By leveraging neural networks in speech recognition and natural language processing, businesses can automate responses, route inquiries more effectively, and cut down on training and labor costs.
The implementation of AI-based voice systems not only improves efficiency but also increases scalability. Call centers can manage a larger volume of inquiries without a proportional increase in staffing. These systems can operate 24/7, ensuring that customers receive timely assistance without the additional overhead of human labor during off-hours.
Key Benefits of Automated Voice Processing
- Cost Efficiency: Reduced need for human agents, especially for repetitive and simple queries.
- Faster Response Times: AI systems can process and respond to inquiries instantly, eliminating wait times.
- Scalability: Easily handles fluctuating call volumes without requiring more staff.
- 24/7 Availability: Provides uninterrupted service regardless of time or day.
How Automation Cuts Costs
"Automated systems allow businesses to reallocate resources to more complex tasks, reducing labor costs while maintaining high customer satisfaction."
- Reduced Training Costs: AI voice systems do not require ongoing training like human agents, which can be expensive and time-consuming.
- Lower Staffing Requirements: The need for additional agents during peak times is minimized, reducing the overall workforce size.
- Minimized Human Error: Automation decreases the chances of costly mistakes or inconsistencies in customer service.
Cost Comparison: Traditional vs. Automated Systems
Aspect | Traditional Call Center | AI-Driven Call Center |
---|---|---|
Agent Salaries | $200,000 per year | $50,000 per year (for maintenance and updates) |
Training Costs | $10,000 per agent | $0 |
Operational Hours | 8 hours a day | 24/7 |
Leveraging Neural Networks for Multilingual Voice Synthesis
Neural networks are increasingly being used to build advanced multilingual voice synthesis systems. These systems leverage deep learning models to generate human-like speech in multiple languages, enabling a seamless experience for global users. By training on large datasets that encompass diverse linguistic features, neural networks can adapt to various phonetic nuances, accents, and intonations across languages.
One of the major advantages of using neural networks in multilingual voice synthesis is their ability to produce natural-sounding speech that mimics human patterns of communication. Unlike traditional methods, which often struggle with the complex rules of different languages, neural networks offer flexibility and precision, facilitating smooth transitions between languages without compromising voice quality or clarity.
Key Features of Multilingual Voice Systems
- Language Adaptability: Neural networks can switch between languages within the same conversation, handling multilingual input with ease.
- Pronunciation Accuracy: Advanced models can mimic the unique pronunciation rules of each language, ensuring proper articulation.
- Contextual Understanding: These systems can adjust their tone, pitch, and cadence depending on the context of the conversation, improving overall communication.
Challenges and Solutions
- Data Scarcity: Some languages have limited voice data available, which can affect the quality of synthesized speech. Solution: Data augmentation techniques and transfer learning are used to overcome this issue by adapting models trained on high-resource languages to underrepresented ones.
- Accent and Dialect Variability: Accents and dialects introduce additional complexity in voice synthesis. Solution: Fine-tuning the model on region-specific data helps improve pronunciation accuracy.
- Real-Time Performance: Generating multilingual speech in real-time can be computationally expensive. Solution: Optimizing the model architecture and utilizing hardware accelerators like GPUs can speed up the process.
Performance Comparison
Feature | Traditional Approach | Neural Network Approach |
---|---|---|
Naturalness | Less natural, robotic | More human-like, fluent |
Language Coverage | Limited support | Supports a wide range of languages |
Adaptability | Limited to predefined rules | Highly adaptable to diverse languages and accents |
Neural networks offer a scalable solution for creating multilingual voice systems, which are key to enhancing user interaction in global markets. With continued advancements in AI, these systems will become even more proficient at understanding and speaking different languages, bridging communication gaps across the world.
Monitoring and Updating Your AI Voice Model for Optimal Performance
Maintaining and improving the performance of your neural network voice model requires ongoing monitoring and timely updates. As AI voice models are influenced by both data and computational resources, it’s crucial to regularly assess their output to ensure they meet the expected quality standards. Monitoring the model’s behavior will help identify any performance degradation and provide insights into areas that require improvement.
Updating your AI voice model is equally important, as the model’s effectiveness can decrease over time due to evolving language use, user preferences, or environmental factors. Regular updates help the model stay relevant, improve accuracy, and adapt to new contexts. This process involves both retraining the model and fine-tuning it based on feedback and performance metrics.
Effective Strategies for Monitoring and Updating
- Track Model Accuracy: Continuously assess the precision of the AI voice by comparing its output against human-generated voice samples.
- Log User Interactions: Collect data from real-world usage to identify patterns, errors, and feedback that can guide improvements.
- Implement Feedback Loops: Use user ratings and direct feedback to improve the model’s responses and adjust it based on changing needs.
- Evaluate Language Use: Periodically update the model with new speech data to ensure it understands current trends and linguistic changes.
Key Metrics to Monitor
Metric | Description |
---|---|
Response Accuracy | Measures how closely the generated voice matches the intended message. |
Naturalness | Assesses the fluidity and realism of the AI’s speech output. |
Latency | Monitors the time taken for the AI to process and generate a response. |
User Satisfaction | Evaluates how well the model’s output meets user expectations based on feedback. |
Key Considerations for Updates
- Data Quality: Ensure the dataset used for updates is diverse, representative, and high-quality to avoid bias in the AI’s responses.
- Computational Resources: Evaluate whether the existing infrastructure supports the scale of retraining required.
- Impact Assessment: Before deploying an update, assess how changes will affect the overall user experience and performance.
- Continuous Testing: Run A/B testing and simulate real-world scenarios to validate the improvements.
Regular monitoring and updates ensure that the AI voice model adapts to new challenges and stays aligned with user expectations. Without these actions, performance could stagnate, and the model may become less effective over time.