Generative Ai and Financial Services
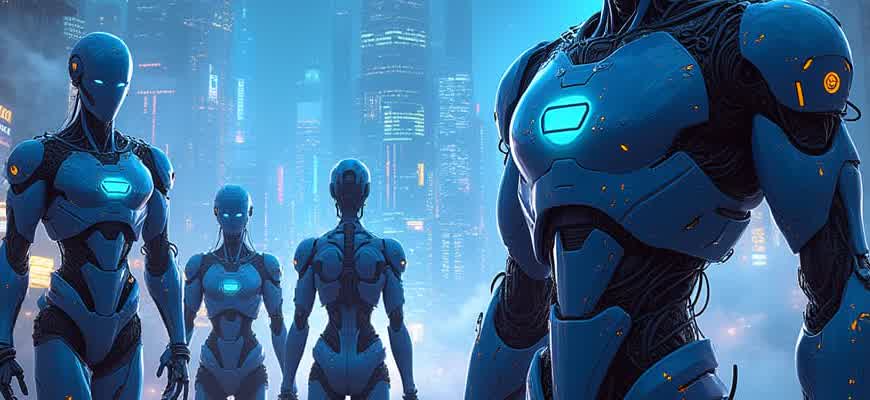
The rise of artificial intelligence technologies is reshaping the financial sector, offering significant improvements in efficiency, decision-making, and customer experience. AI-driven tools, especially generative models, are playing an essential role in automating and optimizing critical processes within banks, investment firms, and insurance companies.
One key area where generative models are making an impact is in predictive analytics. These models can process large volumes of data to forecast market trends, detect anomalies, and assist in risk management. The ability of AI systems to generate insights from vast datasets allows financial institutions to gain a competitive edge by making more informed, timely decisions.
- Improved Risk Assessment: AI tools can generate predictive models that enhance risk analysis and mitigate potential losses.
- Personalized Financial Products: Generative AI can help design custom investment strategies and insurance plans tailored to individual needs.
- Fraud Detection: By analyzing transaction patterns, generative AI models can identify fraudulent activities before they cause significant damage.
"Generative AI is not just an enhancement for financial services; it's a fundamental shift in how businesses approach risk management, customer service, and operational efficiency."
In addition to these practical applications, the integration of AI into financial platforms has also led to more streamlined customer interactions. Chatbots, powered by natural language processing (NLP), are increasingly common in providing instant support, while AI algorithms help personalize user experiences based on individual preferences and behaviors.
Application | Impact |
---|---|
Fraud Detection | Reduces financial losses and enhances security measures by identifying suspicious patterns. |
Customer Service | Improves response times and satisfaction through AI-driven chatbots and personalized support systems. |
Investment Management | Enables the creation of bespoke investment portfolios based on AI-generated insights. |
Generative AI in Financial Services: Unlocking New Opportunities
Generative AI is transforming the landscape of financial services by enabling more personalized, efficient, and data-driven solutions. Financial institutions are increasingly leveraging AI to create tailored products, automate routine tasks, and enhance decision-making processes. With its ability to analyze vast amounts of data and generate actionable insights, AI opens the door to opportunities that were previously unattainable.
From risk management to customer engagement, generative AI is reshaping how financial organizations operate. The technology’s capacity to process and simulate financial scenarios allows firms to predict market movements, optimize portfolios, and reduce potential losses. Moreover, AI-generated content is streamlining communication strategies, providing enhanced customer experiences through personalized interactions.
Key Applications of Generative AI in Finance
- Risk Management: AI models can simulate various financial scenarios to assess potential risks and optimize decision-making processes.
- Personalized Financial Products: By analyzing customer data, AI can design bespoke financial offerings, such as investment strategies, loan options, and insurance policies.
- Fraud Detection: Generative models can identify unusual patterns in transaction data, allowing for quicker detection of fraudulent activities.
- Customer Service Automation: AI-powered chatbots and virtual assistants provide real-time support and enhance customer engagement.
Challenges to Overcome
- Data Privacy Concerns: The collection and use of sensitive customer information require strict security measures to avoid breaches.
- Regulatory Compliance: Adapting AI systems to comply with evolving financial regulations can be a complex task for organizations.
- Bias and Fairness: Generative models need to be carefully monitored to ensure that their outputs do not unintentionally perpetuate biases.
AI-Generated Insights: Real-World Impact
Application | Impact |
---|---|
Risk Forecasting | AI can predict market trends with greater accuracy, helping firms adjust their strategies proactively. |
Portfolio Optimization | Generative models can create personalized portfolios, maximizing returns while minimizing risks. |
Customer Support | AI-driven virtual assistants reduce response time, leading to higher customer satisfaction and engagement. |
"Generative AI is not just a tool for automation; it’s a game-changer in transforming how financial services operate and deliver value to customers."
How Generative AI Improves Risk Management in Financial Institutions
Generative AI is transforming how financial organizations manage risk by leveraging advanced algorithms to predict, simulate, and mitigate potential threats. Through its capacity to analyze vast amounts of data in real time, AI can identify hidden patterns and potential vulnerabilities that traditional systems may overlook. This proactive approach enables firms to react faster and more accurately to market fluctuations, fraud, and regulatory changes.
One of the key advantages of generative models is their ability to simulate different financial scenarios and assess the impact of various risk factors. By continuously learning from new data, AI systems can improve their predictive capabilities, helping institutions to not only detect but also prevent potential risks before they materialize. This enhances both operational efficiency and the safety of financial assets.
Key Areas of Risk Management Enhancement
- Fraud Detection and Prevention: AI systems can analyze transaction data patterns to identify anomalies that may indicate fraudulent activity, thereby reducing the risk of financial loss.
- Market Risk Forecasting: By simulating different economic conditions and market trends, AI can predict the likelihood of significant market changes, giving firms the tools to adjust their strategies accordingly.
- Regulatory Compliance: Generative models can assist in monitoring regulatory changes and ensuring that financial institutions stay compliant, avoiding potential fines or legal issues.
How Generative AI Works in Risk Assessment
- Data Collection and Analysis: AI systems aggregate data from multiple sources–financial reports, market trends, social media sentiment, and historical transaction records.
- Scenario Generation: Based on this data, AI generates possible future scenarios, from market crashes to sudden shifts in consumer behavior, predicting their potential impacts.
- Continuous Learning: As new data becomes available, the model refines its predictions, improving the accuracy of risk assessments over time.
Generative AI empowers financial institutions to anticipate potential risks before they manifest, significantly reducing reaction time and improving decision-making in high-stakes environments.
Example of Risk Management in Action
Risk Type | AI Application | Outcome |
---|---|---|
Market Volatility | Simulating market scenarios under varying economic conditions | Enhanced risk mitigation strategies and better-informed trading decisions |
Credit Risk | Predicting customer default probabilities using past behavior data | Improved lending decisions and reduced non-performing loans |
AI-Based Market Prediction Models in Financial Services
In the evolving landscape of financial markets, predicting market trends in real time has become a critical component for both investors and financial institutions. Traditional methods of market analysis often struggle to process the vast volumes of data in a timely and effective manner. In contrast, AI-driven algorithms are designed to analyze data rapidly, adapt to changing market conditions, and provide highly accurate forecasts. These algorithms leverage machine learning and deep learning techniques to identify patterns in market behavior that might otherwise go unnoticed by human analysts.
One of the most powerful aspects of AI-powered market prediction tools is their ability to perform real-time analysis, using large datasets that include historical prices, economic indicators, social media sentiment, and even geopolitical news. By continually refining their models through feedback loops, these systems become increasingly accurate over time. Financial services companies are now adopting AI models to optimize investment strategies, risk management, and even to predict market downturns before they occur.
Key Components of AI-Driven Market Prediction Algorithms
- Real-Time Data Processing: AI systems process vast amounts of data instantaneously, identifying trends and anomalies that can impact market behavior.
- Machine Learning Models: Algorithms improve their predictive capabilities over time by learning from previous market outcomes.
- Sentiment Analysis: AI models scan social media, news outlets, and other digital platforms to gauge market sentiment, which is a powerful predictor of stock performance.
- Risk Assessment: AI tools predict potential risks, helping firms to minimize exposure and maximize return on investments.
"Real-time market predictions powered by AI not only enhance accuracy but also enable faster decision-making, which is crucial in high-frequency trading environments." - Financial Tech Journal
Advantages and Challenges of AI in Market Prediction
Advantages | Challenges |
---|---|
|
|
- Real-time predictive capabilities enable financial firms to make faster, more informed decisions.
- Market analysis models continue to evolve and improve, making them a valuable tool for long-term strategic planning.
- AI-driven tools can also reduce human error, ensuring that investment decisions are based on data rather than intuition.
Utilizing Generative AI for Scalable Custom Financial Guidance
The financial services industry is increasingly turning to advanced technologies to provide personalized advice to clients. With the rise of generative AI, institutions can now offer customized recommendations at a scale previously thought impossible. By analyzing vast amounts of client data, these AI systems can generate tailored insights that cater to the specific needs and preferences of individual customers, allowing financial advisors to move beyond generic advice.
At scale, generative AI transforms the delivery of financial planning. Through continuous learning, AI can adapt and refine its suggestions based on evolving market conditions and changing personal circumstances, providing clients with up-to-date, relevant financial strategies. This approach not only enhances customer satisfaction but also optimizes operational efficiency by automating many processes traditionally reliant on human advisors.
Key Benefits of AI-Driven Personalized Financial Advice
- Efficiency: AI can quickly process and analyze large datasets, offering rapid insights and recommendations to clients without delay.
- Scalability: Financial institutions can provide personalized advice to a large client base, overcoming the limitations of traditional one-to-one advisory models.
- Customization: AI can factor in a client’s financial goals, risk tolerance, and even behavioral patterns, providing highly specific guidance tailored to the individual.
Operational Framework for Scaling AI-Generated Advice
- Data Collection: Comprehensive and accurate data gathering from multiple touchpoints is essential for training AI systems to offer relevant advice.
- AI Model Development: Developing advanced machine learning models that can understand complex financial situations and generate actionable insights.
- Client Interaction: AI-driven platforms or chatbots that serve as the interface for clients to receive ongoing, dynamic advice.
"Generative AI doesn’t replace human advisors; it enhances their ability to deliver valuable, personalized advice to a broader audience more efficiently."
Example of AI-Generated Financial Advice Platform
Feature | Description |
---|---|
Risk Assessment | AI evaluates a client's financial risk tolerance by analyzing spending habits, investment history, and market trends. |
Goal-Oriented Planning | Generative AI offers specific financial goals (retirement, education, home purchase) with step-by-step investment strategies. |
Continuous Adaptation | The system updates recommendations based on real-time market changes, ensuring advice remains relevant and timely. |
Reducing Fraud in Banking with AI-Powered Anomaly Detection
In the modern banking environment, the risk of fraud is ever-increasing due to the sophisticated nature of financial crimes. To combat this, financial institutions are turning to AI-driven tools to detect and prevent fraudulent activities. These tools leverage machine learning and data analytics to identify unusual patterns in transactions that may indicate fraud. By continuously analyzing vast amounts of financial data, AI can pinpoint anomalies in real-time, providing an effective safeguard against financial crimes.
AI models are trained to recognize normal transaction behaviors and then flag anything that deviates from these patterns. The key advantage is the ability to learn and adapt over time, improving the accuracy of fraud detection. This proactive approach enables banks to respond to potential threats faster, minimizing financial losses and enhancing security for customers.
How AI-Powered Anomaly Detection Works
- Data Collection: Banks gather large amounts of transactional data from various sources, including online banking, ATMs, and mobile apps.
- Model Training: AI models are trained on historical data to understand normal transaction behaviors and relationships between various factors.
- Anomaly Identification: Once deployed, the AI system scans ongoing transactions for irregularities, flagging anything that falls outside expected patterns.
- Real-Time Alerts: When an anomaly is detected, the system generates alerts for investigation, enabling faster response times.
"AI-driven anomaly detection not only catches fraud faster but also reduces false positives, allowing banks to focus their resources on the most critical issues."
Benefits of AI-Powered Fraud Detection
- Faster Response: AI systems can detect and report suspicious activity in real-time, significantly reducing response times.
- Enhanced Accuracy: With continuous learning, AI models improve their ability to distinguish between legitimate transactions and fraudulent ones.
- Cost Reduction: By automating fraud detection, banks can reduce the cost of manual oversight and investigations.
- Scalability: AI systems can handle vast amounts of data, making them ideal for large-scale operations.
Challenges to Consider
Challenge | Impact |
---|---|
Data Privacy | Ensuring that AI systems comply with privacy regulations while processing sensitive financial data. |
False Positives | AI may flag legitimate transactions as fraudulent, leading to unnecessary investigations and customer dissatisfaction. |
Adapting to New Fraud Techniques | Fraudsters are constantly evolving, and AI systems must be updated regularly to stay effective. |
Automating Financial Report Generation Using Generative AI Tools
Financial reporting traditionally requires a significant amount of manual data entry, analysis, and document creation, which can be both time-consuming and prone to human error. However, with the advancements in generative AI, financial institutions now have the opportunity to streamline this process, improving accuracy, efficiency, and consistency in reporting. Generative AI tools, such as natural language processing (NLP) and machine learning models, enable automation of report generation by processing large datasets and transforming them into readable, insightful financial reports.
By leveraging these AI technologies, financial services can automate repetitive tasks, reduce operational costs, and deliver more timely insights to stakeholders. The role of human professionals becomes more focused on decision-making and strategy, while AI handles the data crunching and reporting. This shift not only accelerates the reporting process but also enhances the overall quality and transparency of financial statements.
Key Benefits of Using Generative AI for Financial Report Automation
- Time Efficiency: Automating report generation speeds up the entire process, reducing the time required to produce financial documents.
- Improved Accuracy: AI eliminates human errors associated with manual data entry, resulting in more reliable reports.
- Cost Reduction: By automating repetitive tasks, businesses can reduce the need for manual labor and decrease operational costs.
- Consistency: Generative AI ensures uniformity across multiple reports, adhering to regulatory requirements and company standards.
How Generative AI Transforms the Report Creation Process
- Data Collection and Analysis: Generative AI can quickly gather and analyze large amounts of financial data from various sources, including transactional data, market trends, and economic indicators.
- Report Drafting: Once the data is processed, the AI generates structured drafts, including key performance metrics, balance sheets, and income statements, tailored to the desired format.
- Content Refinement: AI tools can refine the language, ensuring clarity and conciseness while presenting financial insights in a professional tone.
- Compliance Checks: AI models can cross-check the generated content against regulatory frameworks, ensuring that all necessary disclosures and requirements are met.
"Generative AI empowers financial institutions to automate complex report generation, leading to faster decision-making and more accurate financial insights."
Example of Financial Report Automation
Step | AI Contribution |
---|---|
Data Gathering | AI collects data from various systems, including ERP, financial software, and external market databases. |
Report Generation | The AI drafts the report, including key sections like balance sheets, income statements, and cash flow analysis. |
Review and Refinement | AI refines the language, ensuring clarity, and performs compliance checks based on regulatory guidelines. |
Final Report Delivery | The AI outputs the final report, ready for distribution to stakeholders, with reduced errors and optimized formatting. |
How Generative AI Enhances Client Support in Financial Services
Generative AI has the potential to transform customer service in financial institutions by automating repetitive tasks and providing personalized solutions. It allows financial companies to respond to client inquiries quickly and effectively, improving customer satisfaction. This technology aids in addressing complex questions, solving problems, and offering advice in real-time, reducing the dependency on human agents for routine interactions.
By integrating natural language processing and machine learning models, AI systems can understand and generate responses tailored to individual customer needs. These innovations help financial institutions streamline their operations, lower operational costs, and improve efficiency, all while maintaining high levels of customer engagement.
Key Benefits of Generative AI in Financial Customer Service
- 24/7 Availability: AI-driven systems can provide round-the-clock support, addressing customer inquiries at any time without waiting for human agents.
- Personalized Assistance: By analyzing customer data, AI can deliver personalized financial advice and solutions suited to individual needs.
- Faster Response Time: AI minimizes wait times by instantly responding to customer queries, ensuring a smoother experience.
- Scalability: AI systems can handle a large volume of inquiries simultaneously, enabling financial institutions to scale their customer service without significant cost increases.
How It Works: A Practical Example
- Data Collection: The AI system collects data from client interactions, including past inquiries, transaction history, and customer profiles.
- Natural Language Understanding: The system processes the data and understands the intent behind customer queries, even when phrased in different ways.
- Response Generation: Based on the data, the AI generates a tailored response, providing a quick solution or directing the customer to further resources if needed.
Generative AI can significantly reduce human error by providing consistent and accurate responses to customer queries, ensuring clients receive reliable information every time.
Performance Comparison: AI vs. Traditional Support
Aspect | AI-Driven Support | Traditional Support |
---|---|---|
Response Speed | Instant responses to inquiries | Varies depending on agent availability |
Cost Efficiency | Lower operational costs | Higher costs due to human resource requirements |
Availability | 24/7 availability | Limited to business hours |
AI’s Impact on Compliance and Reporting in Financial Services
The application of artificial intelligence in financial institutions has expanded beyond customer service and predictive analytics to critical areas such as regulatory compliance and reporting. With increasingly complex regulations, financial organizations must adopt advanced tools to meet legal obligations efficiently. AI-driven solutions streamline compliance processes, ensuring organizations remain aligned with industry standards while reducing human error and operational costs.
By automating routine tasks such as data entry, transaction monitoring, and the preparation of reports, AI plays a crucial role in enhancing the accuracy and speed of compliance functions. Moreover, AI algorithms can be employed to monitor and predict regulatory changes, enabling organizations to stay ahead of potential compliance issues. This proactive approach helps mitigate risks and avoid penalties associated with non-compliance.
Key Benefits of AI in Regulatory Compliance and Reporting
- Efficiency in Document Processing: AI reduces the manual effort involved in processing regulatory documents, extracting necessary information, and ensuring it complies with specific regulatory frameworks.
- Real-Time Monitoring: AI systems can continuously track financial transactions in real-time, flagging any suspicious activity for immediate review, thereby enhancing anti-money laundering efforts.
- Predictive Analytics: AI tools predict potential areas of concern, allowing firms to act swiftly to adapt to regulatory changes or address compliance risks.
AI-Powered Compliance Reporting Workflow
- Data Collection: AI tools gather data from various sources, ensuring completeness and accuracy in compliance reporting.
- Data Analysis: AI systems process the data, identifying any discrepancies or issues that need addressing.
- Report Generation: AI generates reports automatically, adhering to the format and requirements set by regulatory bodies.
- Audit Trail Creation: AI ensures that every step in the compliance process is documented, creating an unalterable audit trail.
Example of AI Use in Financial Compliance
Task | AI's Role |
---|---|
Transaction Monitoring | AI systems detect unusual patterns in transactions that could indicate fraud or money laundering. |
Risk Assessment | AI evaluates risk levels by analyzing historical data and predicting potential compliance violations. |
Regulatory Reporting | AI automates the creation of reports for submission to regulatory authorities, ensuring accuracy and compliance. |
AI's integration into regulatory compliance and reporting is a game changer for financial services, enhancing operational efficiency, reducing human errors, and ensuring adherence to the ever-evolving regulatory landscape.