Generic Ai Voice
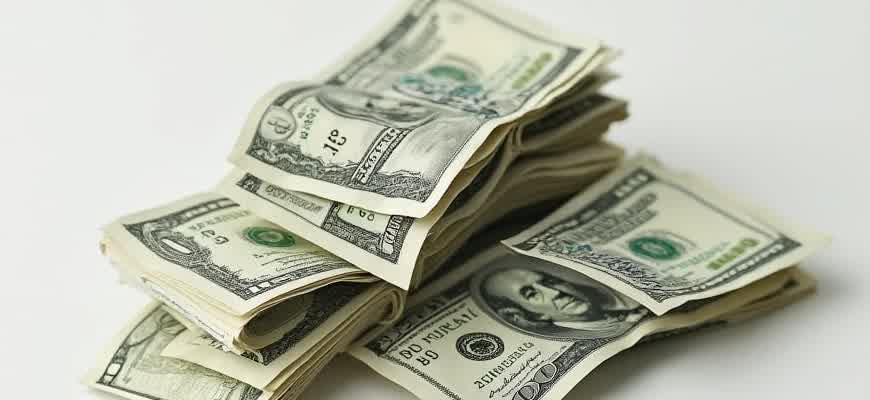
Modern voice synthesis technology powered by deep learning models enables machines to produce human-like speech with remarkable accuracy. These systems can replicate intonation, emotion, and accent, making them indistinguishable from real voices in many contexts.
- Context-aware tone modulation
- Accent and dialect replication
- Real-time voice generation
Voice models trained on diverse datasets can adapt to various communication scenarios, from customer support to virtual assistants, without predefined scripts.
Engineered speech solutions offer scalable alternatives to traditional voice-over work, reducing time and cost across industries such as entertainment, education, and accessibility services.
- Input text is analyzed for emotional cues.
- Voice parameters are dynamically adjusted.
- Speech is generated with phonetic precision.
Feature | Benefit |
---|---|
Neural prosody control | Enables expressive and natural intonation |
Multilingual support | Expands reach across global audiences |
Ways to Customize Voice Tones and Styles to Match Brand Personality
Aligning a synthetic voice with a brand’s unique character requires more than selecting a generic preset. Specific adjustments to rhythm, articulation, and emotional inflection are key to creating a voice that resonates with target audiences. These modifications ensure consistency across customer touchpoints and reinforce brand identity at a subconscious level.
Technical parameters such as pitch, pace, and timbre can be calibrated to reflect values like professionalism, warmth, or innovation. By leveraging advanced AI tools, brands can train models to emulate ideal speaker profiles or mimic spokespersons. This enables seamless integration of voice across channels from virtual assistants to promotional videos.
Key Techniques for Voice Personalization
- Pitch modulation: Lower tones suggest authority; higher tones can indicate friendliness or energy.
- Speech tempo: A slower pace feels thoughtful and premium, while faster delivery fits dynamic, youthful brands.
- Intonation shaping: Varying emphasis adds personality–whether confident, calming, or playful.
Fine-tuning expressive parameters is not optional–it's essential for establishing emotional connection with listeners.
- Define your brand's vocal archetype (e.g., mentor, entertainer, expert).
- Select voice models that align with tone traits such as calmness, clarity, or enthusiasm.
- Customize delivery through API-accessible settings in your TTS engine.
Brand Trait | Suggested Voice Quality |
---|---|
Luxury | Slow tempo, low pitch, crisp articulation |
Youthful | Fast pace, mid-high pitch, energetic tone |
Reliable | Steady rhythm, neutral tone, clear enunciation |
Compliance Considerations When Deploying AI Voice in Regulated Industries
Integrating synthetic voice technologies into sectors like finance, healthcare, or legal services requires strict attention to regulatory frameworks. These environments demand transparency, accountability, and secure handling of sensitive data, making the implementation of voice-driven AI systems particularly challenging. Regulatory non-compliance can result in legal action, financial penalties, and severe reputational damage.
To ensure responsible deployment, organizations must embed governance protocols directly into AI voice workflows. This includes rigorous data anonymization, real-time monitoring, and clear audit trails. Voice interactions must be logged and traceable, with access restricted to authorized personnel only. AI-generated content must not misrepresent human agents or offer advice outside legal or professional scopes.
Key Areas of Compliance Focus
- Data Protection: Adherence to data privacy laws like GDPR or HIPAA is non-negotiable.
- Consent Management: Users must be informed and give explicit consent for voice interactions.
- Transparency: Synthetic voice systems must disclose their non-human nature during engagements.
Failure to disclose AI use in customer interactions may be classified as deceptive practice under consumer protection laws.
- Review applicable regulatory standards by industry and jurisdiction.
- Implement role-based access controls for recorded conversations.
- Establish periodic compliance audits for AI voice system performance.
Industry | Primary Regulation | Compliance Requirement |
---|---|---|
Healthcare | HIPAA | Voice data encryption and patient consent |
Finance | FINRA/SEC | Conversation logging and call monitoring |
Legal Services | ABA Model Rules | Confidentiality and unauthorized practice safeguards |
Cost Breakdown: Automated Voice Systems vs. Human-Operated Call Centers
When evaluating the financial aspects of automated voice systems powered by artificial intelligence compared to traditional support teams, the difference in operational expenses is significant. AI-driven voice agents operate around the clock without requiring salaries, benefits, or breaks, reducing both fixed and variable costs.
Traditional customer support centers incur higher expenditures due to staffing, training, infrastructure, and ongoing human resource management. These factors make them considerably more expensive, especially for businesses scaling globally or dealing with high call volumes.
Key Cost Components
Category | AI Voice Platform | Human Call Center |
---|---|---|
Monthly Operational Cost | $1,000 – $5,000 | $30,000 – $150,000 |
Staffing & Training | None | High |
Scalability | Instant, on-demand | Slow, resource-heavy |
Availability | 24/7/365 | Limited shifts |
Important: AI voice platforms can handle up to 90% of routine queries autonomously, reducing human intervention and increasing cost-efficiency.
- No overtime pay or employee benefits
- Zero cost for breaks, absenteeism, or turnover
- Fixed pricing per call or minute, predictable budgeting
- Deploy an AI voice bot for repetitive tasks
- Route complex issues to a reduced human team
- Monitor analytics to optimize cost-saving opportunities
Strategies for Measuring User Satisfaction After Implementing AI Voice Technology
Understanding how end users respond to newly integrated voice-based systems is crucial for refining their performance. Post-deployment feedback helps identify usability gaps, response accuracy issues, and mismatches in tone or personality of the voice assistant.
To collect meaningful insights, businesses must use structured evaluation models and real-time interaction metrics. These enable ongoing calibration of the voice system's language model, emotional tone, and context handling.
Key Methods for Assessing User Perception
- Surveys with Scenario-Based Questions: Focused questionnaires that ask users to rate their satisfaction based on specific tasks completed using the voice interface.
- Session Analysis: Reviewing audio logs, command repetition frequency, and abandonment rates to detect friction points in interaction flow.
- Post-Interaction Ratings: Requesting quick feedback after each session via simple prompts like “Was this helpful?” with thumb up/down or a star rating.
Systems with more than 3% command repetition rates typically indicate poor intent recognition and reduce user confidence.
- Use A/B testing with different voice personalities to measure emotional response variations.
- Track latency metrics to gauge satisfaction indirectly – delays longer than 2 seconds often correlate with negative user feedback.
- Integrate emotion detection using vocal tone to enrich interaction logs with sentiment data.
Metric | Target Value | Insight Provided |
---|---|---|
Task Success Rate | > 90% | Effectiveness of voice interface in completing user intents |
Average Response Time | < 1.5 sec | User-perceived efficiency of the system |
Sentiment Score | ≥ 0.7 | General tone of user feedback (positive vs negative) |